What Is Multi Agent AI? Vital Things You Need to Know Before Investing
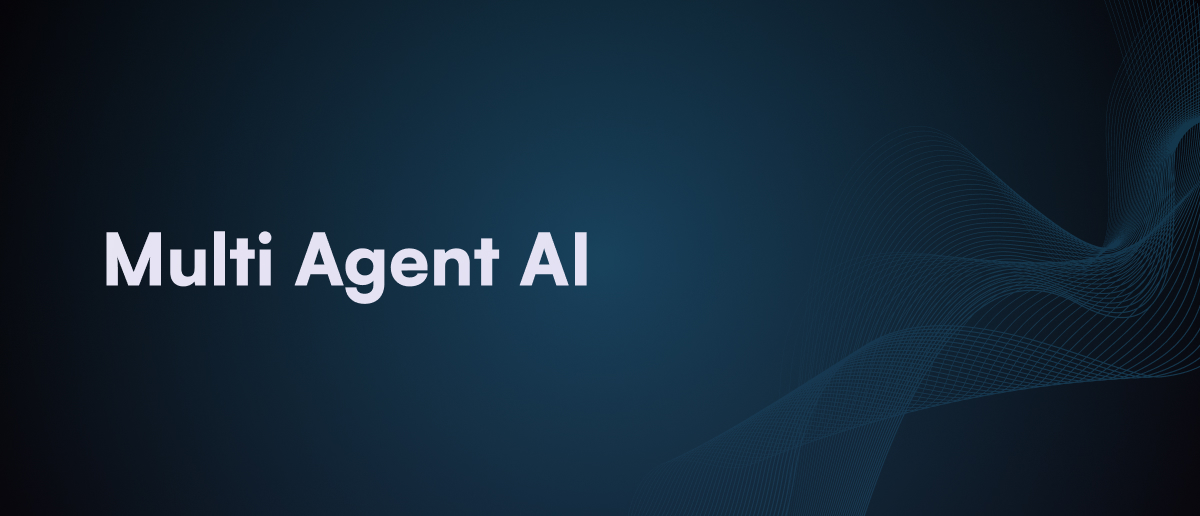
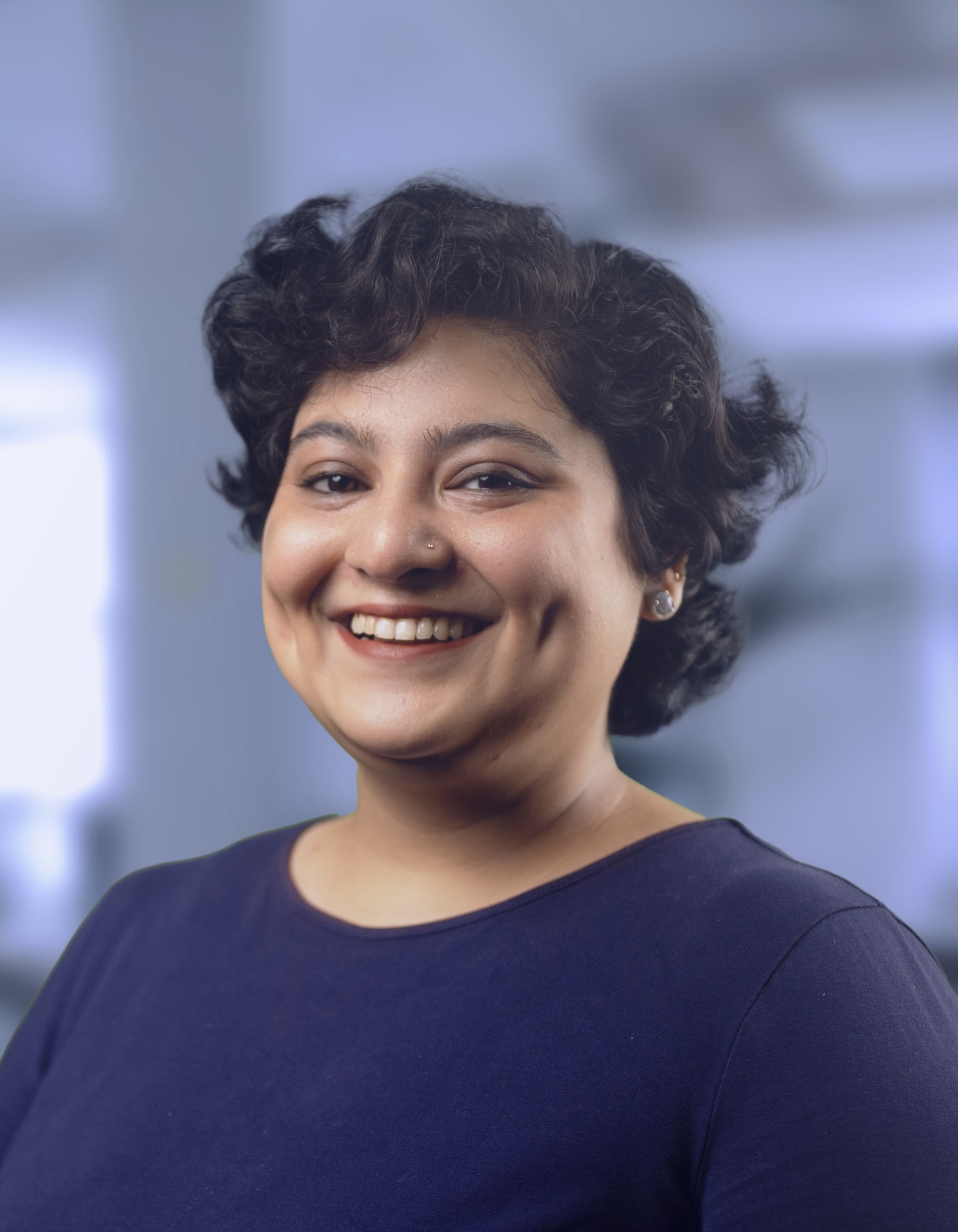
By : Kasturi Goswami
Last Updated: March 12, 2025
12 min read
Table of Contents
Build Your No-Code Custom AI Agent
Get Started FreeAutomation is transforming industries. First, machines replaced manual labor. Then, AI took over repetitive tasks, improving speed and efficiency.
By the early 2020s, businesses started using chatbots for simple automation. This evolved into agentic AI, which later transitioned into single AI agents—handling tasks like customer service, fraud detection, and data processing. These AI models are helpful, but they have clear limitations:
- Limited capacity – A single AI manages multiple tasks but gets overloaded quickly.
- Lack of coordination – Different AI-driven processes remain disconnected.
- Slow adaptation – Single AI agents struggle to adjust to real-time changes.
These inefficiencies create gaps in automation. A single AI cannot handle entire workflows without human intervention. Businesses waste time and resources bridging these gaps manually.
This is why companies are shifting to multi-agent AI. Instead of relying on one AI to do everything, they deploy multiple specialized AI agents. Each AI has a specific role, and together, they communicate, share data, and make intelligent decisions—creating a seamless, efficient AI ecosystem.
What is Multi-Agent in AI?
Multi agent AI is a network of intelligent AIs that collaborate to automate complex workflows. Each AI agent has a specific role but communicates and learns from others.
How do multi agent AIs work?
Multi agent AIs work like a high-performance team and ensure that each part of the system is handled by the most capable AI for the job—creating faster, smarter, and self-improving automation.
By leveraging machine learning, predictive analytics, and reasoning, multi-agents AI:
✔ Continuously learn from new data to improve decision-making.
✔ Share information in real-time to optimize workflow efficiency.
✔ Automate complex processes that require multiple interdependent actions.
Unlike a single AI model, which tries to handle everything with limited flexibility, multi-agents AI divide, conquer, and optimize in real-time.
Core components of a multi agent AI system
- Agents - These are the specialized AI models, each designed for a specific task such as customer service, fraud detection, logistics, or marketing optimization.
- Environment - The ecosystem where AI agents operate, pulling data from various sources such as CRM systems, transaction logs, supply chains, or real-time customer interactions.
- Interactions - AI agents communicate and share insights through structured protocols, enabling coordinated decision-making and task execution.
- Organization - Agents can function under structured rules (hierarchical AI workflows) or use self-organizing strategies, adjusting roles and priorities as needed.
What is the Difference Between a Single-Agent and a Multi-Agent AI System?
As clear as it is, a single-agent system has limitations-operates independently and focuses on one task at a time. A multi-agent system on the other hand consists of multiple AI agents collaborating and exchanging information, making it more dynamic, scalable, and efficient for complex operations.
Let’s understand the above question with a basic example of a multi agent AI system.
Imagine running an e-commerce business. A single AI chatbot might manage customer service, but with multi-agent AI, you get a powerhouse system:
✔ Customer AI – Answers inquiries, recommends products.
✔ Inventory AI – Tracks stock levels, adjusts supply chains.
✔ Marketing AI – Tweaks ad campaigns in real time.
✔ Pricing AI – Monitors competitor pricing and adjusts dynamically.
These agents communicate and self-optimize, so when one detects a spike in demand, the others respond instantly—adjusting prices, updating ads, and restocking inventory without human intervention.
To understand the multi-agent AI architecture better, we suggest you go through this conceptual video by LangChain.
The shift from a single-agent system to a multi-agent system removes bottlenecks, speeds up processes, and makes automation smarter. Businesses are quickly shifting from a single AI that struggles under pressure. Instead, they have a system that adapts, scales, and continuously improves.
The future of AI isn’t one model doing everything. It’s many AI agents working together—efficient, intelligent, and built for growth.
Single-Agent System Vs. Multi-Agent System
Factor | Single-Agent System | Multi-Agent System |
---|---|---|
Behavior | Operates independently with a centralized control mechanism. | Functions as a distributed system, where multiple agents coordinate and collaborate to achieve goals. |
Architecture | Typically follows a monolithic design, where a single AI model handles all tasks. | Follows a modular and decentralized design, where multiple AI agents perform specialized roles. |
Communication | Works in isolation; does not interact with other AI models or agents. | Uses agent-to-agent communication via message-passing protocols, shared databases, or APIs. |
Learning | Uses supervised or unsupervised learning, often trained as a single model with static knowledge. | Incorporates reinforcement learning, federated learning, or self-learning mechanisms where agents continuously improve. |
Complexity | Lower computational complexity; requires less memory and processing power. | Higher complexity due to distributed coordination, real-time decision-making, and inter-agent communication overhead. |
Control & Autonomy | Centralized control where the AI follows predefined logic and does not deviate from its assigned task. | Decentralized control, where agents make independent decisions while collaborating dynamically with other agents. |
Scalability | Limited scalability; becomes inefficient with increasing task complexity. | Highly scalable, as new agents can be added or removed without affecting overall system performance. |
Context Awareness | Limited or non-existent; decisions are made based on immediate inputs with no memory of past interactions. | Uses contextual reasoning and historical data to make decisions, improving accuracy over time. |
Decision-Making | Follows linear or tree-based decision-making (rule-based, deterministic). | Uses multi-layered decision-making, where agents adapt based on environmental feedback and real-time interactions. |
Adaptability | Rigid and static; cannot easily adjust to dynamic environments. | Adaptive and flexible; can respond to unexpected events, market changes, and user behavior shifts. |
Fault Tolerance | Low fault tolerance—if the agent fails, the system breaks down. | High fault tolerance—if one agent fails, other agents continue working, maintaining system stability. |
Dependency | Works independently; no interdependence with other AI models. | Agents rely on interactions with other agents, making them dependent on a coordinated framework. |
Task Allocation | Follows static task allocation; all decisions are made by the central AI. | Uses dynamic task allocation, where agents can take on new roles or redistribute workloads as needed. |
Resource Utilization | Utilizes system resources inefficiently when handling multiple tasks. | Optimizes resource allocation by distributing tasks among specialized agents. |
Use of Distributed AI | Not applicable; operates on a single model or instance. | Uses distributed AI principles, where different AI agents specialize in specific areas and collaborate for holistic problem-solving. |
Computing Infrastructure | Requires less computational power, as it processes tasks sequentially. | Needs high processing capabilities, often using cloud computing or edge computing for real-time collaboration. |
Coordination Mechanism | No coordination is needed; single AI executes tasks independently. | Requires coordination mechanisms like centralized, decentralized, or hybrid control systems to manage interactions between agents. |
Performance in Dynamic Environments | Low adaptability; struggles with real-time updates and complex decision-making. | High adaptability; agents continuously refine strategies based on changing conditions. |
Security Risks | Lower risk, as a single AI model follows a controlled environment. | Higher security concerns, since multiple AI agents exchange data, requiring robust encryption and cybersecurity measures. |
Interpretability & Transparency | Easier to interpret and debug; decisions are based on fixed rules or models. | Harder to analyze, as decisions result from inter-agent collaboration and learning. |
Flexibility in Workflow | Follows predefined workflows; cannot shift roles dynamically. | Allows adaptive workflows, where AI agents can adjust responsibilities as needed. |
Real-World Use Cases |
Key Advantages & Characteristics of Multi Agent AI
- Autonomy & hyper-efficiency - These AI agents operate independently, making instant decisions without centralized control. Businesses gain faster execution and reduced human dependency.
- Collaboration & continuous optimization - Multi agent AI share data, refine strategies, and adjust actions based on collective learning. As they interact, they improve both individually and as a system.
- Real-time adaptability & responsiveness They react instantly to market shifts, customer behaviors, and supply chain fluctuations. Whether it’s a spike in demand or a supply chain disruption, agents coordinate and respond in milliseconds.
- Flexibility & scalability - Businesses can add, remove, or retrain AI agents without disrupting workflows. This means companies can evolve their automation strategies as needs change and markets shift.
- Self-organization & coordination - Instead of requiring manual oversight, multi agent AI prioritize tasks, allocate resources, and resolve conflicts dynamically.
- Robustness & reliability - Even if one AI agent fails or malfunctions, the system adapts and continues operating without breaking down.
How to Implement Multi Agent AI in your Business?
Step 1: Define your business needs
- Identify bottlenecks - where could AI improve speed, efficiency, or accuracy?
- Break down tasks - customer service, analytics, and operations each need a specialized agent.
- Set clear goals - what do you want your AI agents to achieve?
Step 2: Choose an AI agent framework
Not all Multi agent AI systems are the same. Your framework should include:
- Inter-agent communication - AI agents must share real-time insights for smarter decision-making.
- Autonomous learning - Your AI should evolve and optimize itself without constant human intervention.
- Scalability - The system should grow with your business without requiring a full rebuild.
Step 3: Start small & scale
- Deploy a minimum viable product - (MVP) with two or three specialized agents.
- Track performance metrics - where do they excel? Where can they improve?
- Expand strategically - add new AI agents as business needs evolve.
Integrating Multi Agent AI with Modern AI Technologies
Multi agent AI are getting smarter and faster with the latest AI breakthroughs. These technologies help them learn, adapt, and collaborate like never before.
- Large Language Models (LLMs) - Improve reasoning and natural conversations. AI can now understand, summarize, and respond more like humans. What's more, LLMs can even help build multi-agent AIs. Here's how, fresh off from the MLOps Community.
- Reinforcement learning - Helps AI learn from experience. Agents can now optimize decisions and improve over time without human input.
- Neural networks - Enhance pattern recognition and predictions. AI can detect trends, personalize experiences, and make smarter recommendations.
- Blockchain - Enables secure, decentralized AI collaboration. Agents can now share data safely in industries like finance and supply chain management.
- Edge computing - Speeds up real-time AI processing. AI can analyze data instantly, improving self-driving cars, IoT, and automation.
You might also be interested in: 30+ Best AI Tools For Businesses
What Challenges does a Multi Agent AI System Face?
A Multi-agent AI is powerful, but it’s not perfect. Businesses need to consider a few key challenges before diving in.
- Complex setup - Multi-agent systems need a solid AI framework, strong communication between agents, and smooth data integration. That’s not easy to build.
- High computing power - These systems process massive amounts of real-time data. Unlike single AI models, they need more storage, faster processing, and advanced infrastructure.
- Security risks - AI agents constantly exchange data. Without proper encryption and access controls, they could become vulnerable to cyber threats.
- Coordination issues - If agents aren’t properly trained to collaborate, they can make conflicting decisions. This can lead to inefficiencies instead of improvements.
- Ongoing costs - Multi-agent AI isn’t a one-time setup. It needs constant monitoring, updates, and optimization, which can be resource-heavy for smaller businesses.
Despite these challenges, businesses that adopt AI strategically can scale into Multi-agent AI over time. The key is starting with the right framework and building step by step.
Thinkstack AI is the Smartest First Step
Jumping straight into Multi-agent AI can be overwhelming. It needs planning, investment, and expertise. Many businesses aren’t ready for that level of complexity.
That’s where Thinkstack AI makes things easier. It gives you the power of multi-agent AI—without the headache.
Here’s how:
✔ Works with what you have – Seamlessly integrates with WhatsApp, Facebook Messenger, Instagram, Zapier, and more.
✔ Learns and adapts – Gets smarter with every interaction.
✔ Goes beyond chatbots – Thinkstack AI isn’t just a rule-based system. It makes strategic decisions.
✔ No complex setup – Start with smart AI automation. Scale when you’re ready
The best part is that Thinkstack’s AI agents already works like a multi-agent system. It connects workflows, shares insights, and automates decisions—just like a Multi-agent AI system but without the hassle and challenges.
For businesses that want AI without the big investment, Thinkstack AI is the perfect first step. It lets you see AI in action, drive automation, and scale when the time is right.